Introduction
In today’s data-driven world, organizations across industries are recognizing the immense value of big data analytics in making informed, strategic decisions. Big data analytics involves the collection, processing, and analysis of large and complex datasets to uncover insights, patterns, and trends that can drive better decision-making. In this article, we will explore how companies are harnessing the power of big data analytics to make data-driven decisions, the strategies they employ, and the profound impact this approach has on their operations, growth, and competitiveness.
-
Understanding Big Data Analytics
1.1 What is Big Data?
Big data refers to extremely large and diverse datasets that exceed the processing capabilities of traditional data management tools. It encompasses structured data (e.g., databases and spreadsheets), semi-structured data (e.g., XML and JSON files), and unstructured data (e.g., text, images, videos, and social media content).
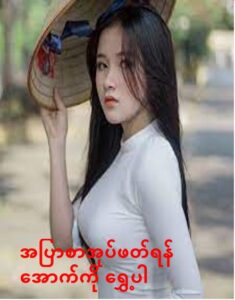
1.2 The Three Vs of Big Data
- Volume: Big data involves vast amounts of data generated at an unprecedented scale. This includes data from sensors, social media, online transactions, and more.
- Velocity: Data is generated and updated at high speeds, requiring real-time or near-real-time processing and analysis.
- Variety: Data comes in various formats, including structured, semi-structured, and unstructured data. It may include text, images, audio, video, and sensor data.
1.3 The Role of Big Data Analytics
Big data analytics involves using advanced technologies and techniques to extract meaningful insights from these large and diverse datasets. This process encompasses data collection, data cleansing, data transformation, data analysis, and visualization.
-
The Value of Data-Driven Decision-Making
2.1 Improved Decision Quality
Data-driven decision-making is based on evidence, analysis, and facts rather than intuition or gut feeling. This approach leads to more informed and objective decisions, minimizing the risk of errors.
2.2 Enhanced Customer Insights
Big data analytics allows companies to gain a deep understanding of their customers’ preferences, behaviors, and needs. This insight enables personalized marketing, product recommendations, and improved customer experiences.
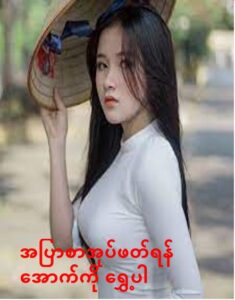
2.3 Operational Efficiency
By analyzing data on processes, supply chains, and operations, companies can identify bottlenecks, inefficiencies, and areas for improvement. This optimization leads to cost savings and improved productivity.
2.4 Competitive Advantage
Organizations that effectively leverage big data analytics gain a competitive edge. They can adapt quickly to changing market conditions, identify emerging trends, and capitalize on opportunities ahead of their competitors.
2.5 Risk Mitigation
Data-driven decision-making helps companies identify and assess risks more accurately. By analyzing historical data and patterns, organizations can make informed decisions to mitigate potential risks and crises.
III. Strategies for Implementing Big Data Analytics
3.1 Data Collection and Integration
Companies need a robust strategy for collecting and integrating data from various sources, both internal and external. This involves identifying relevant data sources, ensuring data quality, and establishing data pipelines.
3.2 Scalable Infrastructure
Scalable infrastructure, including cloud computing and distributed computing frameworks like Hadoop and Spark, is essential for handling the volume and velocity of big data. These technologies enable parallel processing and storage of large datasets.
3.3 Data Warehousing and Data Lakes
Data warehouses and data lakes serve as centralized repositories for structured and unstructured data, respectively. They provide a foundation for data storage, organization, and retrieval.
3.4 Data Governance and Security
Data governance policies and security measures are critical to protect sensitive data and ensure compliance with regulations like GDPR and HIPAA. Companies must establish data access controls, encryption, and auditing mechanisms.
3.5 Advanced Analytics Tools
Selecting the right analytics tools is crucial. Companies use a combination of tools for data preparation, data analysis, and data visualization. Popular choices include Python, R, SQL, and business intelligence platforms.
3.6 Data Science and Analytics Talent
Hiring and nurturing data science and analytics talent is essential. Skilled professionals are needed to develop models, algorithms, and data-driven solutions.
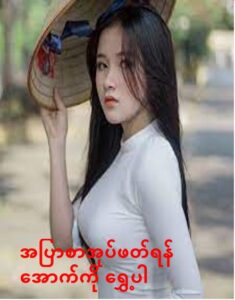
-
Use Cases of Big Data Analytics in Decision-Making
4.1 Retail and E-commerce
- Demand Forecasting: Retailers use big data analytics to predict customer demand accurately, optimize inventory levels, and reduce stockouts or overstocking.
- Customer Personalization: E-commerce platforms leverage data to provide personalized product recommendations, marketing campaigns, and user experiences.
- Price Optimization: Dynamic pricing algorithms adjust product prices based on real-time demand, competitor pricing, and other factors.
4.2 Healthcare
- Patient Care: Healthcare providers use big data analytics to improve patient outcomes by analyzing electronic health records (EHRs) and patient data.
- Disease Prediction: Big data analytics helps in early disease detection and prediction by analyzing patient data and clinical research.
- Drug Discovery: Pharmaceutical companies analyze vast datasets to identify potential drug candidates and streamline the drug discovery process.
4.3 Finance and Banking
- Risk Management: Financial institutions use big data analytics to assess credit risk, detect fraudulent transactions, and monitor market trends.
- Algorithmic Trading: High-frequency trading relies on big data analytics to make split-second trading decisions based on market data and patterns.
- Customer Insights: Banks analyze customer data to identify upselling and cross-selling opportunities, enhance customer experiences, and reduce churn.
4.4 Manufacturing and Supply Chain
- Predictive Maintenance: Manufacturers use IoT data and machine learning to predict equipment failures and schedule maintenance proactively.
- Supply Chain Optimization: Big data analytics optimizes supply chain logistics, reduces transportation costs, and minimizes delays.
- Quality Control: Manufacturers analyze production data to ensure product quality and reduce defects.
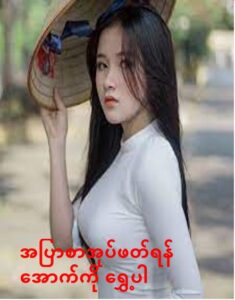
4.5 Marketing and Advertising
- Customer Segmentation: Marketers use data analytics to segment their target audience and tailor advertising campaigns accordingly.
- Ad Campaign Effectiveness: Big data helps evaluate the effectiveness of ad campaigns by tracking click-through rates, conversion rates, and other metrics.
- Social Media Monitoring: Companies analyze social media data to gauge brand sentiment, identify influencers, and respond to customer feedback.
- Challenges and Considerations
5.1 Data Privacy and Security
Companies must prioritize data privacy and security to protect sensitive information and comply with data protection regulations.
5.2 Data Quality
Ensuring data accuracy and quality is crucial for reliable analysis and decision-making.
5.3 Scalability
As data volumes continue to grow, companies need scalable infrastructure and tools to handle large datasets effectively.
5.4 Talent Shortage
There is a shortage of data science and analytics talent. Companies must invest in training and development to bridge this gap.
-
The Future of Big Data Analytics
6.1 AI and Machine Learning Integration
AI and machine learning will play an increasingly significant role in automating data analysis, uncovering deeper insights, and enhancing decision-making.
6.2 Edge Analytics
Edge analytics, which processes data at the source (e.g., IoT devices), will become more prevalent, enabling real-time decision-making without relying solely on centralized data centers.
6.3 Ethical Considerations
Ethical considerations, including bias in algorithms and responsible data use, will gain prominence in big data analytics.
6.4 Democratization of Data
Tools and platforms that democratize data access and analysis will enable non-technical users to harness the power of big data.
Conclusion
Big data analytics is no longer a luxury but a necessity for organizations seeking to remain competitive and make informed decisions in the data-driven era. By adopting robust strategies, investing in talent, and leveraging the right tools and technologies, companies across industries can unlock the transformative potential of big data analytics, leading to improved decision quality, enhanced operational efficiency, and a distinct competitive advantage. As the volume and complexity of data continue to grow, the journey of harnessing big data analytics for informed decision-making is an ongoing and evolving one that will shape the future of businesses and industries worldwide.